Toru ISHIGAMI
Fine Particle Technology Laboratory, Chemical Engineering Program,
Graduate School of Advanced Science and Engineering
E-mail: ishigami_at_hiroshima-u.ac.jp (Please replace "_at_" to "@".)
Key points of this research results
- Machine learning using a convolutional neural network (CNN) as a surrogate model was used to predict the flow field and submicron particle behavior in the air filter microstructure.
- Flow field parameters obtained from computational fluid dynamics (CFD) simulations were used as the training data set for the CNN.
- The flow field predicted by the CNN was in good agreement with that calculated by CFD simulation. A simulation speedup of three orders of magnitude was obtained by CNN for predicting the flow field.
- By coupling the flow field predicted by the CNN with the discrete phase model (DPM), the dynamics of submicron particles in the filter microstructure was successfully calculated.
Outline
Fibrous filters are dust collection devices that captures particles through various mechanisms such as inertial impact, interception, Brownian diffusion, and gravity setting. Despite the well-established classical theory of filtration efficiency for aerosol particles, the performance analysis of realistic filters and microstructure optimization, which comprises a wide variety of different fiber geometries and arrangements, requires high-resolution numerical simulations to predict the details of the fluid-particle dynamics. However, such computational fluid dynamics (CFD) simulations have the disadvantage of being very computationally time-consuming. This study applies machine learning to overcome this drawback.
In this study, a convolutional neural network (CNN), which is used in image recognition and analysis, was used as a surrogate model. For the training data set of the CNN, we used the results of CFD simulations conducted by varying the fiber arrangement and fiber geometry. The velocity and pressure fields around the fibers predicted by the CNN were found to be in good agreement with the CFD simulations conducted under the same conditions. In addition, a simulation speedup of three orders of magnitude was obtained by CNN for predicting the flow field.
The flow field obtained by CNN was coupled to a discrete phase model (DPM) to calculate the dynamics of submicron particles in the filter. Comparison of the collection efficiency obtained by conventional CFD-DPM methods showed generally good agreement, although there was a slight tendency to slightly overestimate the collection efficiencies for particles smaller than 0.3 μm, indicating that the method developed in this study is suitable for fibrous filter design and optimization purposes.
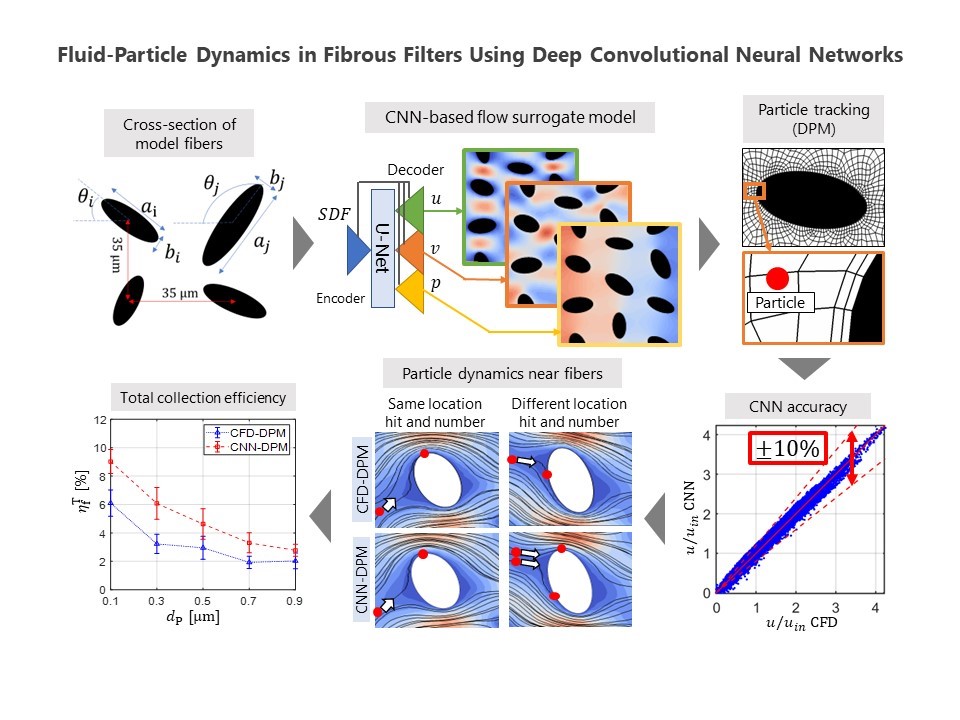
Paper Info
Shirzadi, M., T. Fukasawa, K. Fukui, T. Ishigami : "Application of Deep Learning Neural Networks for the Analysis of Fluid-Particle Dynamics in Fibrous Filters" Chemical Engineering Journal, 455, 140775 (2023). https://doi.org/10.1016/j.cej.2022.140775
Shirzadi, M., T. Fukasawa, K. Fukui, T. Ishigami : "Prediction of Submicron Particle Dynamics in Fibrous Filter Using Deep Convolutional Neural Networks" Physics of Fluids, 34, 123303 (2022). https://doi.org/10.1063/5.0127325