Key points of this research results
- AI (Artificial Intelligence) technology-based method was proposed to identify building damage grades induced by natural disasters from post-disaster aerial images. Collapsed, non-damaged and blue tarp-covered buildings are automatically classified by the proposed method.
- The AI model was developed the aerial images and building damage data obtained in recent earthquakes, tsunami, typhoons, landslides and flood disasters. We confirmed that the building damage in the disasters was accurately classified by the proposed method.
- The proposed method can provide detailed building damage map immediately after natural disasters, and it will contribute for rapid and cost-effective post-disaster responses.
Outline
After large-scale natural disasters such as earthquakes, it is necessary to assess an extent and amount of damage as soon as possible in order to consider appropriate emergency responses. Especially for building damage, it is important to assess not only major damage such as collapsed buildings but also moderate damage in order to identify the extent of the damage. In this study, we proposed an AI technology-based damage identification method using the aerial images and building damage data obtained in recent natural disasters such as earthquakes, tsunami, typhoons, landslides and flooding including the 1995 Kobe, Japan earthquake and the 2016 Kumamoto, Japan earthquake.
Deep learning model was developed for automatically classifying the building damage to collapsed, non-damaged and blue tarp-covered buildings. Convolutional neural network (CNN), one of the deep learning techniques, was applied to the data from the two earthquakes. We confirmed that more than 90 % of the buildings in the validation dataset were accurately classified by the proposed method. We also revealed that the building damage maps visualized from the estimation results would be helpful in understanding the distribution and limits of the building damage in the disasters.
This method can automatically provide the distribution of building damage in a short time, and the estimated damage maps are expected to be used as basic information for early stage post-disaster responses in the future.
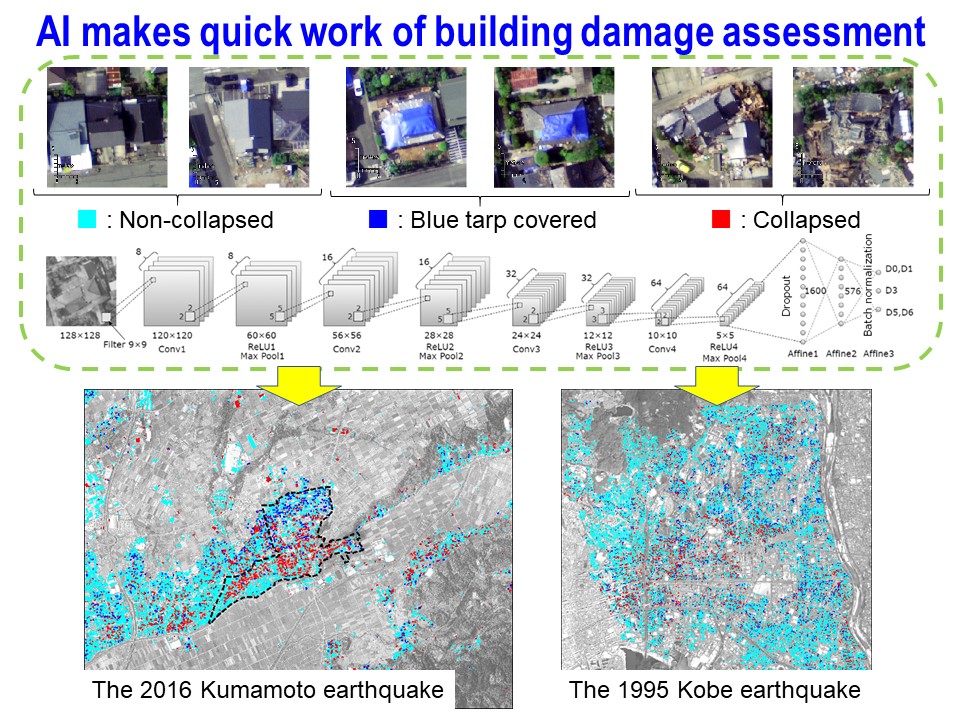