Key points of this research results
- This research concerns adaptive data-sharing methods for multi-agent systems using deep reinforcement learning.
- Three training data-sharing methods are proposed, and simulation experiments are conducted to demonstrate their effectiveness.
- The proposed methods outperform conventional methods and can be tailored to the characteristics of each target problem.
- Experiments using a maze environment showed that the proposed method outperforms existing methods.
- This research's results are expected to improve data-sharing methods in multi-agent systems.
Outline
In multi-agent environments with multiple agents that learn based on reinforcement learning or deep reinforcement learning, it has been reported that information sharing between agents can improve learning efficiency. However, learning efficiency will likely decrease if information sharing is insufficient or excessive. This study proposes three types of training data-sharing methods for multi-agent systems using deep reinforcement learning. Specifically, we constructed three methods: randomly selecting data, ranking data based on reward and sharing a certain percentage, and adjusting the percentage according to the learning status.
In this study, we showed the effectiveness of the proposed methods by conducting simulation experiments using a maze problem. The simulation experiment results showed that the proposed methods outperformed the existing methods and could be adjusted to fit the characteristics of each target problem. This research result is expected to improve data-sharing methods in multi-agent systems. However, there may be some limitations or challenges in the actual application. ;
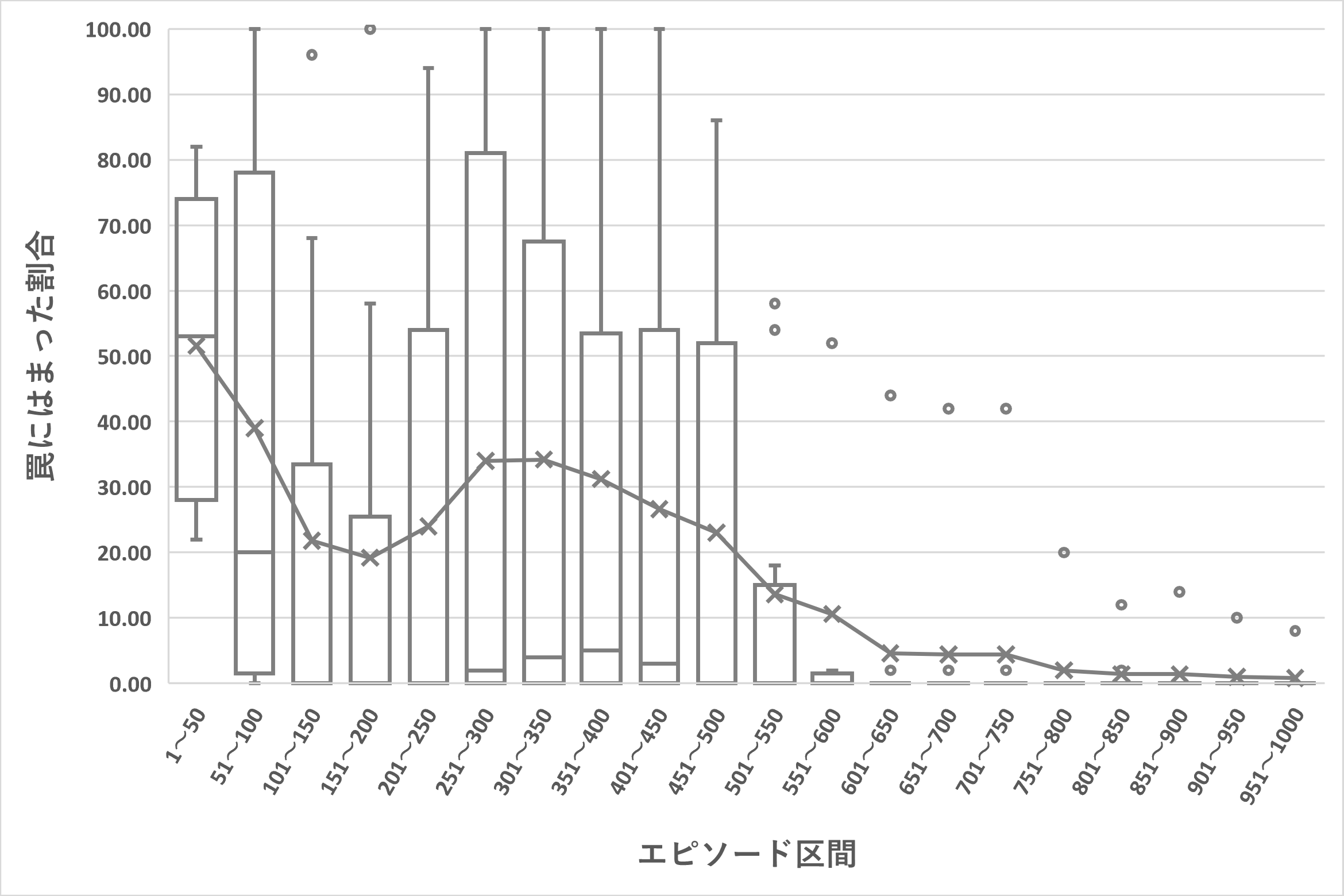
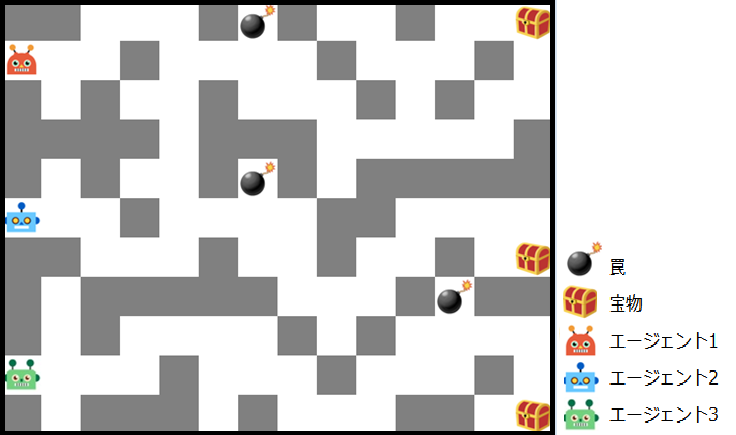