Key points of this research results
- This study proposes a pattern classification method that can decode human motion intentions from electromyogram (EMG) signals generated by muscle contraction.
- The proposed method incorporates a stochastic generative model, and the variance of the EMG signal is treated as a latent random variable based on a stochastic generative model, thereby allowing pattern recognition to consider the uncertainty involved in the signal.
- In the training of the proposed method, approximate inference of the posterior distributions by the variational Bayes and the hyperparameter determination method based on mutual information maximization are introduced to optimize the structure and characteristics of the model according to individual differences in EMG patterns.
- Experiments using multiple EMG datasets showed that the proposed method outperforms general-purpose pattern classifiers in terms of classification accuracy and computational cost.
Outline
Electromyogram (EMG) generated by muscle contraction has been utilized to interface signals for prosthetic hands and information devices owing to its ability to reflect human internal states. To realize such EMG-based applications, it is necessary to recognize human motion intentions from the measured EMG patterns with high accuracy.
However, the variance of EMG signals often involves the fluctuation caused by the uncertainty of muscle activity, and the conventional EMG pattern recognition methods do not fully consider this phenomenon.
In this study, we propose a scale mixture-based stochastic model to represent the uncertainty of EMG signals and apply it to EMG pattern recognition. In the proposed method, the EMG variance is handled as a latent random variable, which can represent the uncertainty depending on the muscle activity. In addition, a learning algorithm based on variational Bayesian inference is adopted, thereby allowing the automatic determination of the model complexity during the training process. To optimize the hyperparameter included in the proposed method, a determination method based on mutual information maximization is introduced.
These learning strategies allow us to optimize the structure and characteristics of the model according to individual differences in EMG patterns. In the experiments, the classification accuracy of the proposed method was verified using several EMG datasets. The results revealed that the proposed method outperformed the various conventional general-purpose classifiers.
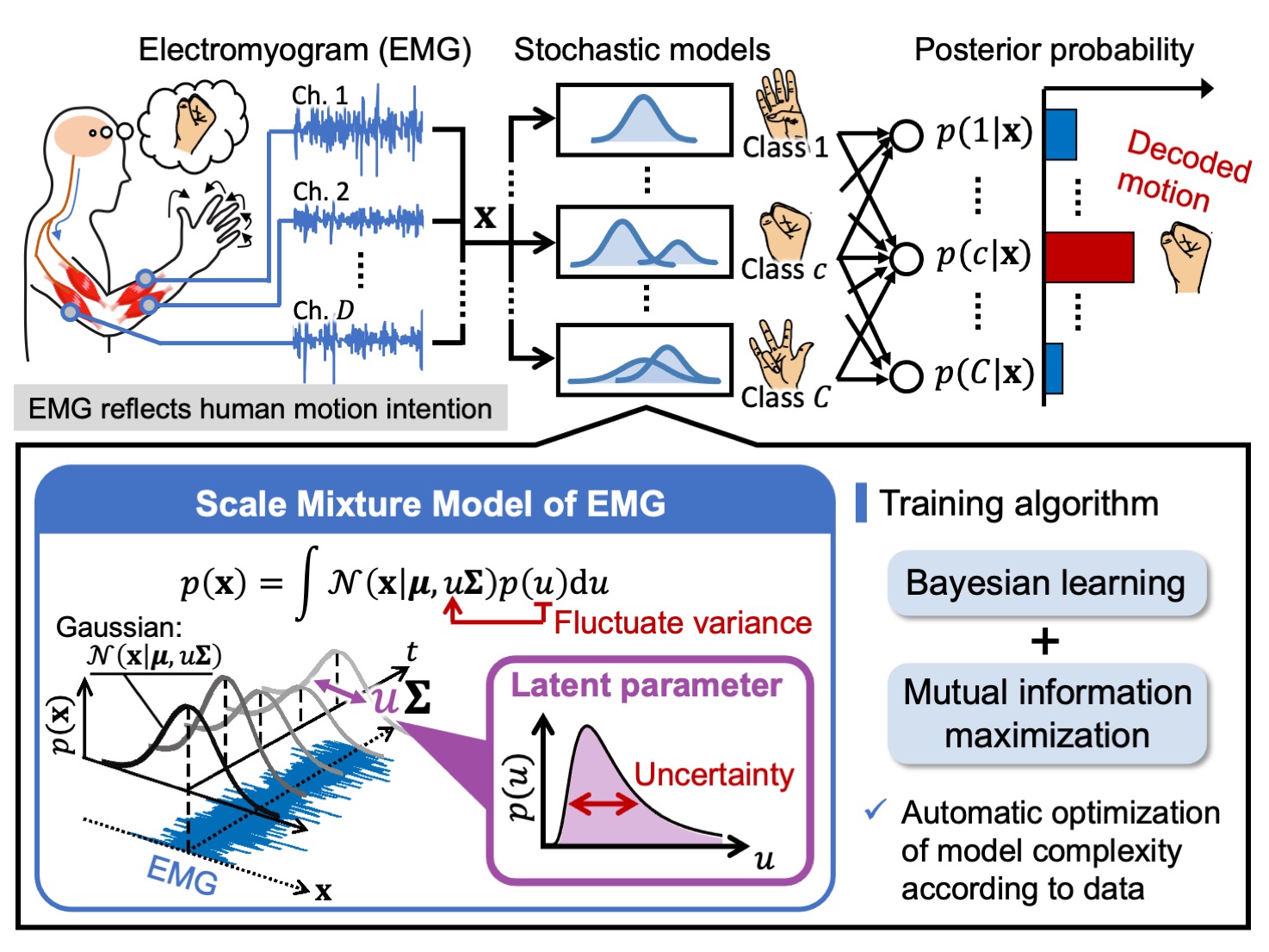