Kazuhiko, Hayakawa. Recent Development of High-Dimensional Time Series Analysis.Journal of the Japan Statistical Society Japanese Issue. 2014, Vol.43, No.2, p.275-292, 03895602.
‘Big Data’ is collected and accumulated in various situations by businesses and economists and used to conduct high-level analyses. This paper looks at how this Big Data is analyzed so it can be used for macroeconomic forecasts such as for business conditions and inflation. Recently, business condition forecasts have become more accurate through analyzing the tens to hundreds of various types of high-dimensional macro time-series data. This paper introduces the statistical methods used to analyze such large numbers of data variables.
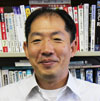
Kazuhiko Hayakawa
Graduate School of Social Sciences, Professor
Research Fields: Social sciences;Economics;Economic statistics
Presently, the development and spread of information and telecommunication technologies has made it possible to collect and accumulate a great deal of data automatically from various situations. Such data, known as Big Data is for example much sought after by the business world for the beneficial information it contains. Big Data is also utilized in economics. For example, there are studies on the creation of new price indices using the point of sales (POS) data of supermarkets. Also, in the field of quantitative finance, stock and foreign exchange data in units of seconds and minutes, referred to as tick data, are used.
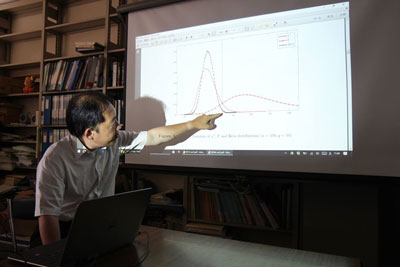
This study introduces several examples of research into the many different types of macro time-series data. One theme reoccurring in macroeconomics, is the issue of measuring business conditions. The basic approach until recently has been to select three or four units (e.g., production index, ratio of job offers to job seekers etc.) of representative economic data and to analyze them using statistical and econometric methods. However, the choice of variables then is dependent on the subjective view of the analyst, and a lingering issue has been the variation in results depending on such chosen variables. This problem is attributed to the shortcomings of traditional statistical methods: e.g. their use is limited to the analysis of a small number of variables as it is difficult to employ them to analyze large numbers (high-dimensional data). However, the development of statistical methods that allow for the analysis of high-dimensional data in recent years has made it possible for us to use such methods to measure business conditions using, tens or even hundreds of various types of high-dimensional macro time-series data. An example exists in Europe, where the Centre for Economic Policy Research (CEPR) publishes an economic index created using high-dimensional macro time-series data referred to as EuroCOIN.
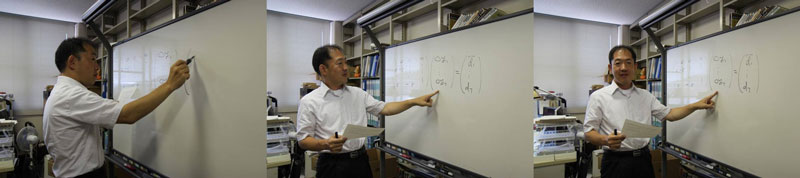
Quantitative analysis using such high-dimensional data is currently a central theme in econometrics, with various studies being undertaken. For example, when predicting the inflation rate, it has been demonstrated that the prediction based on high-dimensional data is far more accurate than that based on traditional methods. The development of such statistical methods for analyzing high-dimensional data is thus a central theme in this study.
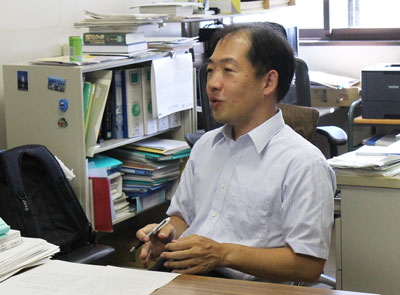